The 2024 Data Guide for Customer Experience Leaders
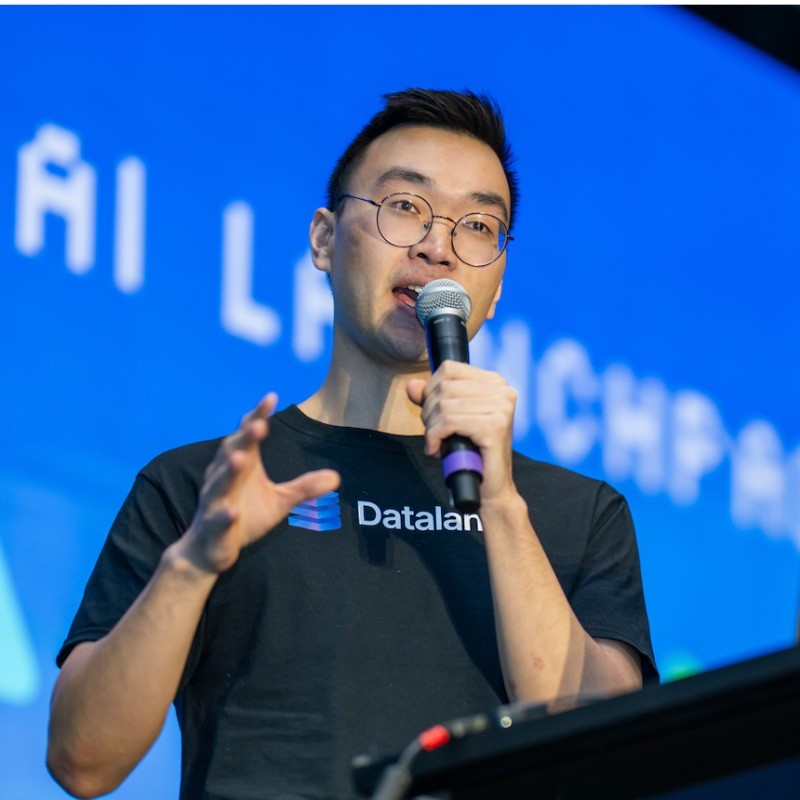
When it comes to scaling customer experience, data plays a central role. It's not just about tracking metrics or reporting. The real challenge is building data infrastructure and tooling that evolves with your team's needs.
In this guide, we'll cover:
- How to recognize where your CX data maturity currently stands
- What strategies, tools, and processes are needed based on maturity stage
- Tips on how to advance in CX data maturity
This post is not about metrics or KPIs to memorize. This post is about how to benchmark your CX team's data maturity, and how to strategically set up your data landscape for scaling CX through the next 1-3 years – from perspectives of leaders that have scaled from Series A to startup exit and beyond.
First, what is data maturity, and why does it matter for CX?
Data maturity is a helpful way to think about "what good looks like" and where your CX team's approach to data sits in that spectrum. Otherwise, it's too easy to waste time on boil-the-ocean approaches which typically cripple data initiatives.
In this guide, we break down what the levels of maturity are, what's exciting / challenging in each level (so you know what to watch out for), and how to tactically progress to the next one (and who you'll need to talk to along the way).
Stage 1: Data Silos
Stage 1 is about having disconnected data silos for earlier-stage CX teams (Seed to Series A). There might only be a handful of customer support specialists. For B2B companies, knowledge about key accounts can sufficiently stay tribal, so there's little value in investing in data infrastructure for morecomplex workflows.
Stage 1 indicators
Data team size: There is no data staff dedicated to the CX team. Total company data team is fewer than 5 people.
Data integration: Data is scattered across lots of different places, like the core product database + application data, billing systems like Stripe, CRM data (Hubspot, Salesforce), transactional messaging touchpoints (Klaviyo, Customer.io, SendGrid), and helpdesk data (Zendesk, Intercom).
Seeing all relevant data points about a single customer requires accessing each one of these different SaaS applications. Customer support agents need to open 5+ tabs to different data systems for each customer issue. Internal databases are also hard to check without having engineering build internal tools or database viewers on it.
Seeing broader patterns across data sources is impossible without one-off engineering help or lots of spreadsheet cobbling – e.g. "how often are customers in X state that have ordered product Y filing support tickets"
When to evolve past Stage 1
- Customer support teammates start to complain about the "number of clicks" that it takes to resolve customer issues.
- Due to data sprawl, average handle time (AHT) starts to become unsustainably high. This starts to threaten customer satisfaction (CSAT). Ultimately, this could drive greater headcount due to inefficient tooling.
- Customer success is based on general "vibes" and anecdotal notes from recurring meetings, but lack of engagement (which could have been realized in the data) can cause nasty surprises when customers decline to renew contracts.
How to evolve past Stage 1
Start gathering information on what parts of your CX team's workflows are inefficient. Specifically - ask your team to keep track of how many minutes and clicks it takes to handle 10-20 common issues for customer support. To make the case:
- Tie inefficiency to headcount needs, AHT, CSAT, and ultimately retention issues (anecdotal evidence can be very powerful here)
- Identify the most common data sources used, and point to the financial + team morale impact of improving tools such that teammates don't have to waste time on repetitive clicks.
- For customer success, work with product and engineering to set up analytics dashboards that report customer-level behavior at a glance. Product / engineering teams are also incentivized to evaluate the success of their own work, and will often touch much of the same data that CX needs.
Possible solutions + tools:
- Show custom data fields in customer details in helpdesk software.
- Adopt data syncing tools like Zapier or use pre-built integrations over the systems you control
- Build simple dashboards for easier “data lookup” on customer information, using tools like Metabase or Tableau
- Adopt a customer success platform with basic health score implementation
Stage 2: The Duct Tape Phase
Stage 2 is what I call the "duct tape phase" - you've recognized the problems of disconnected data and started patching things together, but in an ad-hoc way that won't scale. This is where most companies get stuck at the Series A - D stages. You might have a customer success platform that shows health scores, and your support team might have some basic dashboards about high-level metrics, but it's all held together with metaphorical duct tape and prayer.
Stage 2 Indicators
Partial integration: You've connected some systems - maybe Zendesk shows basic Shopify data, or your CSP pulls in some usage metrics. But it's incomplete and inconsistent due to data quality issues (like duplicate rows, missing values, or wrong values).
Inability to deep dive: Let’s say that a CSM gets alerted to a poor health score. The health score may/may not be accurate, but they’ll need to deep dive into customer behavior to understand the ground truth. A CSM might ask, “Customer X isn’t engaged, but has customer X trying out feature Y yet?” “Has the customer set up the necessary integration to do that feature”? “And stepping back – are there other customers that have similar behavior?"
This process of doing a deep dive – and then zooming out to verify – can’t be done with static dashboards of CSPs that prevent the CX team from doing their own analyses.
CX team has grown, but still no dedicated data resource: Your CX team has grown significantly. You've added specialists for CX ops, voice of customer programs, and consumer insights. But your data team is still small (usually under 10 people) and stretched thin.
Data ability gap: A few "data heroes" on your team can dig deep and create insights, but most CX folks still struggle to answer their own questions.
Tool Sprawl: You're adding new tools faster than you can integrate them - new payment processors, partner platforms, marketing tools. Each adds another layer of complexity.
"“I need my CSMs to have a clearer picture of what’s going with their accounts. What sort of technical issues are they running into? Are they using a certain feature? How much?
We have Salesforce, Catalyst, and Tableau, but it’s not enough to just have top-level dashboards. But my CSMs need to be proactively investigating data on their own, but our current tooling makes that too difficult.
— VP of Customer Success, Series C
The Chicken-and-Egg Problem
The biggest challenge at this stage is breaking out of the "chicken-and-egg" problem when getting the data team to help. The data team doesn't prioritize CX because they don't see enough value from working on CX data initiatives in isolation, but CX also can't demonstrate value because they lack proper data infrastructure. So the data team keeps serving finance and executive reporting, while CX struggles with band-aid solutions.
This manifests in many small inefficiencies:
- CSMs can't easily drill down from high-level health scores to understand what's actually happening
- Support reps waste precious minutes jumping between tools
- Manual processes that worked at a smaller scale start breaking down
- Data quality issues crop up because information is being passed through too many systems
How to advance to Stage 3
The key insight is that you can't scale by just adding more connections between systems. If you try to sync everything to everything else, you'll create a nightmare of data inconsistency. Instead:
- Build the case for data centralization: Document exactly how much time your team wastes gathering data. Multiply that by your headcount and growth projections. The numbers usually shock executives into action.
- Start with the end in mind: Map out your ideal customer data model. What would you need to know about each customer to provide amazing service? This becomes your north star.
- Invest in a proper data foundation: You need a central place where all customer data lives and updates in real-time. This isn't just another tool - it's core infrastructure.
- Empower the front lines: Give CX teams the ability to answer their own questions. This might mean training, better tools with a friendly learning curve, or ideally both.
To accomplish this, CX teams adopt tools like Dataland.io, which centralizes data from disconnected sources into one place, in real-time, and also enable CX teams to search, analyze, and ask AI to get answers they need from customer data.
Common mistakes
"We have 30+ dashboards and counting in Metabase. It was ok at first but they're getting slow, hard to maintain, and are a big drag on the team. Every new feature our product team launches means that we have to ask the data team to build one more dashboard. Adding more dashboards is not a solution in the limit."
— Senior Director of Global Support, Series C
- Building more and more dashboards: The key realization is that your CX will always have data needs that pop up at the margins. Asking the data team to build another ad-hoc dashboard is not sustainable. At a certain point - the dashboard sprawl also unwieldy and confusing for CX operators – making it difficult to onboard new agents without significant ramp-up time.
- Centralizing data in a data warehouse: The data team’s first inclination is to centralize data into a data warehouse like Snowflake or BigQuery. This is often the correct choice for finance teams that need to report on general metrics over long periods of time. However, data warehouses run at an hourly data refresh schedule at most, and typically run on daily intervals. This means that key data for time-sensitive workflows in CX (especially in support) won’t be available.
Stage 3: Data Maturity (But Don't Get Comfortable)
Stage 3 is when things start working smoothly - but paradoxically, this is also when you're still vulnerable. The biggest mistake companies make at this stage is thinking they've "solved" their data problems.
How to tell if you're stage 3
- Data Quality: Your customer data is centralized, clean, and trustworthy
- Tool Efficiency: CX teams have mature dashboards and can self-serve most of their data needs
- Process Maturity: Clear workflows exist for handling data needs and changes
Stage 3 is an equilibrium - not a destination
Stage 3 isn't a destination - it's a sensitive balance that's disrupted by:
- Entering new markets with different data requirements
- Acquisitions that bring in new data models
- Product launches that challenge your existing data model
- Regulatory changes that force architectural updates
Staying at Stage 3
The key to maintaining Stage 3 maturity is building flexibility into your foundation:
- Design for change: Your data architecture should expect and welcome new sources and requirements.
- Maintain strong partnerships: Keep close relationships between CX, data, and engineering teams. The moment these groups stop communicating regularly, problems start growing.
- Regular health checks: Don't wait for problems to surface. Regularly audit your data quality, tool usage, and team efficiency.
- Invest in documentation: As systems mature, tribal knowledge becomes a liability. Document not just what exists, but why decisions were made.
"Working with Dataland has been nothing short of phenomenal. We just acquired a competitor earlier this year with its own data systems and software. The Dataland team has taken all of our combined, complex data to create a tool that enables our reps, broader CX org, and whole company to answer data questions on their own.
— Senior Director of Global Support, Series C
The most successful companies at Stage 3 paradoxically spend less time talking about data and more time using it to solve customer problems. When your data foundation is solid, it becomes invisible infrastructure that just works.
Remember: The goal isn't perfect data - it's having data mature enough to scale your customer experience effectively. Focus on progress over perfection, and build systems that can evolve with your needs.